Parallel Ultra-Low Power Computing for Extreme Edge AI – A RISC-V Open Platform Approach
Luca Benini
ETH Zürich and University of Bologna
October 20, 2020
▸ Video Presentation: 4PM Lisbon / 8AM California / 11 PM Beijing
▸ Questions & Answers (Live): 4:45PM Lisbon / 8:45AM California / 11:45 PM Beijing
Abstract: Edge Artificial Intelligence is the new megatrend, as privacy concerns and networks bandwidth/latency bottlenecks prevent cloud offloading of sensor analytics functions in many application domains, from autonomous driving to advanced prosthetic. The next wave of “Extreme Edge AI” pushes signal processing and machine learning aggressively towards sensors and actuators, opening major research and business development opportunities. In this talk, I will focus on recent efforts in developing an AI-centric Extreme Edge computing platform based on open-source, parallel ultra-low power (PULP) RISC-V processors coupled with domain-specific accelerators.
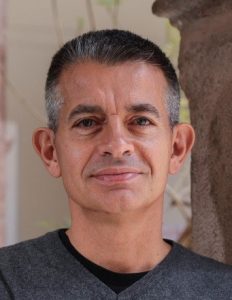
Biography: Luca Benini holds the chair of Digital Circuits and Systems at ETHZ and is Full Professor at the Universita di Bologna. He received a PhD from Stanford University. He has been visiting professor at Stanford University, IMEC, EPFL. In 2009-2012 he served as chief architect in STMicroelectronics, France. Dr. Benini’s research interests are in energy-efficient computing systems design, from embedded to high-performance. He is also active in the design of ultra-low-power VLSI circuits and smart sensing micro-systems. He has published more than 1000 peer-reviewed papers and five books. He is an ERC-advanced grant winner, a Fellow of the IEEE, of the ACM and a member of the Academia Europaea. He is the recipient of the 2016 IEEE CAS Mac Van Valkenburg award and the 2020 ACM/IEEE A. Richard Newton Award.
How to Evaluate Efficient Deep Neural Network Approaches
Vivienne Sze
MIT
October 21, 2020
▸ Video Presentation: 4PM Lisbon / 8AM California / 11 PM Beijing
▸ Questions & Answers (Live): 4:45PM Lisbon / 8:45AM California / 11:45 PM Beijing
Abstract: Enabling the efficient processing of deep neural networks (DNNs) has becoming increasingly important to enable the deployment of DNNs on a wide range of platforms, for a wide range of applications. To address this need, there has been a significant amount of work in recent years on designing DNN accelerators and developing approaches for efficient DNN processing that spans the computer vision, machine learning, and hardware/systems architecture communities. Given the volume of work, it would not be feasible to cover them all in a single talk. Instead, this talk will focus on *how* to evaluate these different approaches, which include the design of DNN accelerators and DNN models. It will also highlight the key metrics that should be measured and compared and present tools that can assist in the evaluation.
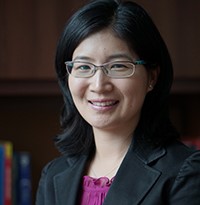
Biography: Vivienne Sze is an associate professor of electrical engineering and computer science at MIT. She is also the director of the Energy-Efficient Multimedia Systems research group at the Research Lab of Electronics (RLE). Sze works on computing systems that enable energy-efficient machine learning, computer vision, and video compression/processing for a wide range of applications, including autonomous navigation, digital health, and the internet of things. She is widely recognized for her leading work in these areas and has received many awards, including the AFOSR and DARPA Young Faculty Award, the Edgerton Faculty Award, several faculty awards from Google, Facebook, and Qualcomm, the 2018 Symposium on VLSI Circuits Best Student Paper Award, the 2017 CICC Outstanding Invited Paper Award, and the 2016 IEEE Micro Top Picks Award. As a member of the JCT-VC team, she received the Primetime Engineering Emmy Award for the development of the HEVC video compression standard. She co-author of the recent book entitled “Efficient Processing of Deep Neural Networks” (Morgan & Claypool, 2020).
More from the Energy-Efficient Multimedia Systems Group at MIT
5G and Intelligent Wireless Systems
Shilpa Talwar
Intel
October 22, 2020
▸ Video Presentation: 4PM Lisbon / 8AM California / 11 PM Beijing
▸ Questions & Answers (Live): 4:45PM Lisbon / 8:45AM California / 11:45 PM Beijing
Abstract: Wireless data rates have grown at a predictable cadence every year (roughly 1.5x/year), which is not surprisingly correlated to the growth rate of transistor density as predicted by Moore’s Law (1.6x/year). In this presentation, we will discuss the fundamental technologies that have led to this exponential data rate scaling from 2G-to-5G, and identify what to expect with future rate scaling and technical limits. 5G standard has also represented a fundamental shift from 1G-4G, moving from human-centric communications, to ‘connectivity of Things’. The connectivity requirements of machines/IOT devices & services vary dynamically, ranging from a massive number of low-rate connections to ultra-reliable low latency connections. In order to meet the demanding requirements of IoT, 5G networks deployments are becoming increasingly dense, hierarchical, and heterogeneous. Moving forward, we expect networks Beyond 5G (B5G) to represent yet another transformative shift, expanding from primarily a connectivity function to enabling the ‘autonomy of Things’. This shift towards autonomy will mandate the integration of new real-time capabilities within the network, such as analytics, learning, decision-making, perception and control. Hence, the ever-increasing network diversity and complexity of emerging services will require us to rethink our system design approach. We will need to take a cross-disciplinary view across Communications, Computing, and Intelligence, and develop fundamental innovations that lie in the intersection of these disciplines. We will share 3 broad research directions that we are pursuing in Intel Labs: a) Intelligence Defined Networking, b) Multi-Access Edge Intelligence, and c) Edge Compute Offloading. We expect these technologies will be essential components of next-generation wireless networks.
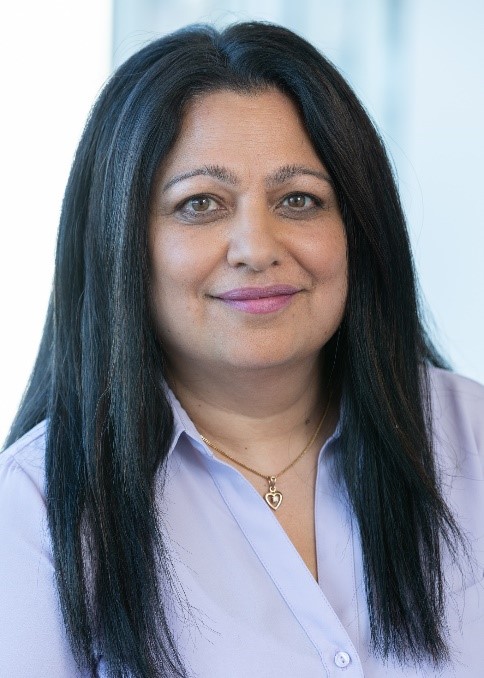
Biography: Shilpa Talwar is an Intel Fellow and director of wireless multi-communication systems in the Intel Labs organization at Intel Corporation. She leads a research team in the Wireless Communications Laboratory focused on advancements in ultra-dense multi-radio network architectures and associated technology innovations. Her research interests include multi-radio convergence, interference management, mmWave beamforming, and applications of machine learning and artificial intelligence (AI) techniques to wireless networks. While at Intel, she has contributed to IEEE and 3GPP standard bodies, including 802.16m, LTE-advanced, and 5G NR. She is co-editor of the book on 5G “Towards 5G: Applications, requirements and candidate technologies.” Prior to Intel, Shilpa held several senior technical positions in wireless industry working on a wide range of projects, including algorithm design for 3G/4G & WLAN chips, satellite communications, GPS, and others. Shilpa graduated from Stanford University in 1996 with a Ph.D. in Applied mathematics and an M.S. in electrical engineering. She is the author of 70 technical publications and holds 60 patents.